The Role of Agriculture Datasets for Machine Learning in Enhancing Business Efficiency
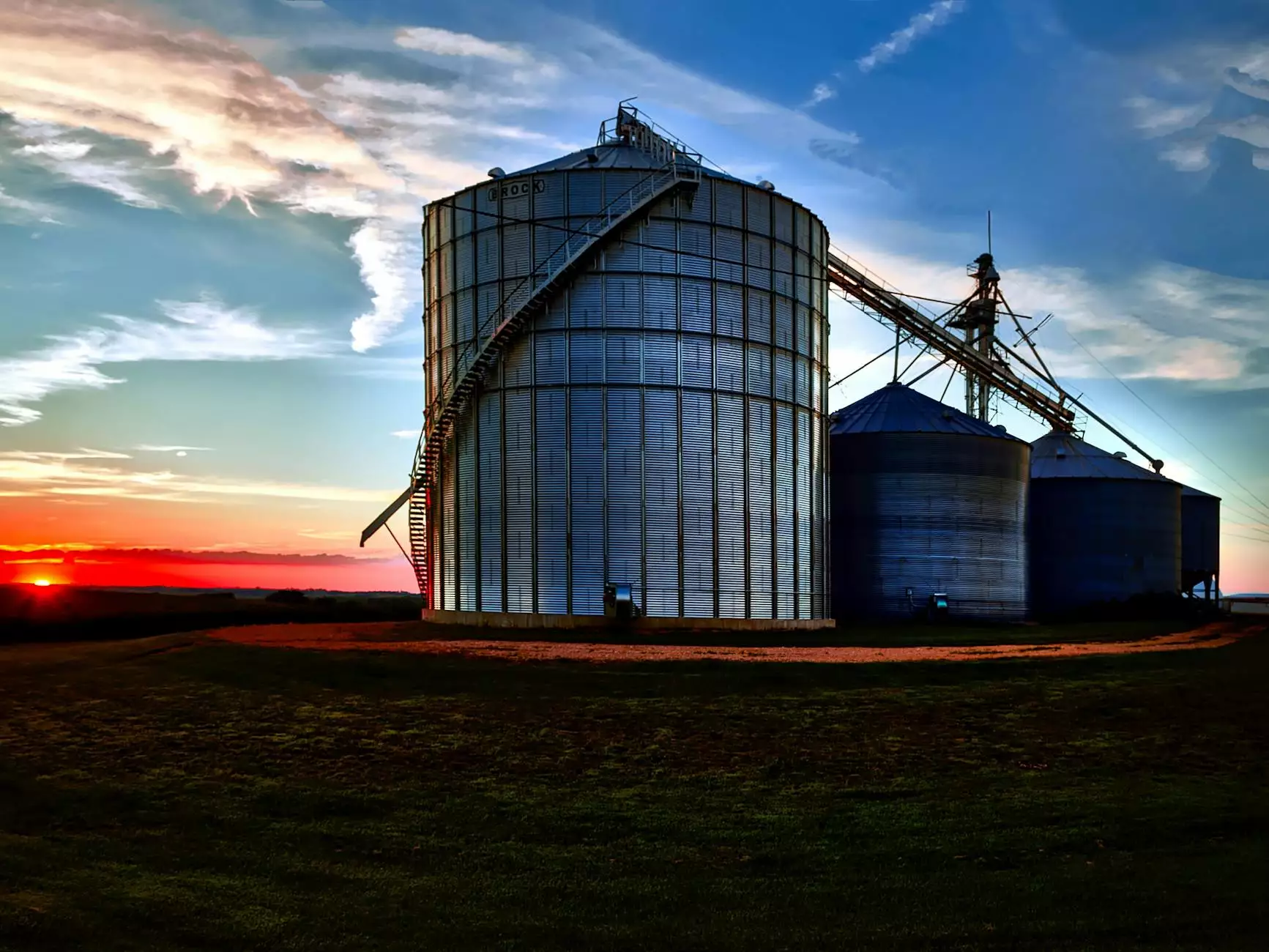
In today’s rapidly advancing technology landscape, the integration of data-driven insights into business practices is crucial. The agricultural sector, in particular, stands to gain immensely from the application of machine learning techniques utilizing vast and detailed agriculture datasets. This article delves deep into how these datasets can fuel smarter decision-making, enhance operational efficiencies, and ultimately transform agricultural businesses into modern, high-performing enterprises.
Understanding Agriculture Datasets
Agriculture datasets are collections of data that encompass various aspects of farming and agricultural practices. They include data related to crop yields, soil characteristics, weather patterns, pest outbreaks, and more. The richness of these datasets provides a foundation upon which machine learning algorithms can analyze trends, identify patterns, and make predictions that are pivotal for effective farming.
Types of Agriculture Datasets
- Crop Yield Data: Information on the productivity of various crops based on diverse conditions.
- Soil Data: Attributes of soil types including nutrient composition, pH levels, and moisture levels.
- Weather Data: Historical and predictive insights into weather patterns.
- Pest and Disease Data: Records of pest occurrences and disease outbreaks, aiding in management strategies.
- Market Data: Information on pricing trends and market demands for various agricultural products.
Leveraging Machine Learning with Agriculture Datasets
Machine learning (ML) has emerged as a vital tool in optimizing agricultural practices, providing farmers and agricultural businesses with actionable insights that lead to enhanced productivity. By leveraging machine learning models on well-structured agriculture datasets, businesses can achieve unprecedented levels of efficiency. Here’s how:
1. Precision Farming
Through precision farming, farmers can optimize field-level management with regard to crop farming. By utilizing agriculture datasets for machine learning, farmers can:
- Analyze Soil Variability: Understand the different properties of soil across a farm to apply fertilizers and water more efficiently.
- Target Pest Management: Use timely insights from data analysis to apply pest control measures only where necessary.
- Optimize Crop Rotation: Use predictive modeling to decide the best crops to plant based on historical yield data.
2. Enhanced Decision-Making
The decision-making process in agriculture can be significantly enhanced through the utilization of data analytics. By implementing machine learning algorithms that analyze vast databases, agricultural businesses can:
- Forecast Yields: Predict agricultural output based on historical trends and environmental data.
- Optimize Resource Allocation: Allocate resources such as water, fertilizers, and labor more effectively to improve efficiency and reduce costs.
3. Risk Management
Certain risks in agriculture, such as climate change and pest losses, can be mitigated with improved data analysis. Machine learning models can predict adverse conditions, allowing businesses to prepare adequately and make informed decisions to minimize potential losses. This capability leads to a more resilient agricultural sector capable of adapting to unpredictable circumstances.
Benefits of Using Agriculture Datasets for Machine Learning
Employing agriculture datasets in machine learning processes offers numerous benefits that can profoundly influence the operation and profitability of agricultural businesses. Some of these benefits include:
Cost Savings
By maximizing the efficiency of resources—such as inputs, labor, and time—businesses can drastically cut down on operational costs. Machine learning provides companies with the tools to precisely assess their agricultural practices, leading to better resource management through data-informed decisions.
Increased Crop Yields
Properly analyzed data can help predict the most productive planting strategies. By understanding the optimal growing conditions and pest management, farmers can experience enhanced crop yields, contributing to greater profits.
Market Competitiveness
In a global marketplace, the ability to adapt quickly to changing conditions is essential. Utilizing agriculture datasets for machine learning equips businesses with timely insights that can inform marketing strategies, leading to increased market competitiveness and customer satisfaction.
Sustainability
Precision farming enabled by machine learning fosters sustainable farming practices. Efficient resource use minimizes waste and environmental impact, leading towards a greener future for the agriculture sector.
Implementing Machine Learning in Agricultural Practices
To successfully implement machine learning techniques using agriculture datasets, businesses need to take the following steps:
1. Data Collection
The first step is to gather relevant data. Agricultural businesses should focus on acquiring comprehensive and high-quality datasets. This might involve:
- Partnering with research institutions and agricultural experts for specialized datasets.
- Utilizing sensors and IoT devices to collect real-time farming data.
- Incorporating satellite imagery for extensive analysis.
2. Data Preparation
Once data is collected, it must be adequately processed. This involves cleaning the data to eliminate inconsistencies, transforming data to suit machine learning algorithms, and organizing it into a format that is easily interpretable.
3. Model Development
The choice of machine learning model plays a crucial role in the analysis. Different models, such as regression models, decision trees, and neural networks, can be selected based on specific agricultural objectives. Methods like cross-validation should be employed to ensure model accuracy.
4. Analysis & Interpretation
After the model is developed, it is essential to analyze the outcomes. Insights gained from the model will inform actionable strategies that agricultural businesses can implement.
5. Continuous Improvement
The agricultural landscape is continually changing; thus, businesses must routinely revisit their datasets and models to ensure they remain relevant and effective. Continuous learning and adaptation are key to maintaining competitive advantages.
Challenges in Utilizing Agriculture Datasets for Machine Learning
Despite the numerous advantages, there are challenges that agricultural businesses may encounter, including:
Data Quality and Availability
Accessing high-quality and relevant datasets can be difficult. In some cases, data may be outdated or unavailable, which could affect the reliability of machine learning predictions.
Technological Barriers
Not all agricultural businesses are equipped with the necessary technology or infrastructure to implement machine learning solutions efficiently. Investments in technology and training may be required.
Interpretation of Results
Data analysis through machine learning can be complex, and wrong interpretations can lead to poor decision-making. Therefore, having skilled data scientists is crucial for proper analysis and implementation.
The Future of Agriculture with Machine Learning
The future of agriculture is poised for transformation through the innovative utilization of agriculture datasets for machine learning. As more data becomes available from various sources, the potential for machine learning to optimize agricultural practices will expand. Here are a few trends that could shape the agriculture landscape:
Integration of IoT and AI
The convergence of Internet of Things (IoT) devices and Artificial Intelligence (AI) will enable real-time data collection and analysis. This integration will enhance decision-making and operational efficiencies.
Enhanced Crop Management
Future advancements in ML will allow for even more precise crop management techniques. Farmers will leverage predictive analytics to ensure optimal planting and harvesting periods.
Increased Use of Automation
With the growth of autonomous farming machines and tools often driven by data, the agriculture sector will see increased automation, leading to enhanced productivity and reduced labor costs.
Conclusion
The utilization of agriculture datasets for machine learning signifies a pivotal shift in how agricultural businesses operate. By embracing data-driven decision-making, these businesses can significantly enhance productivity and promote sustainable practices. Investing in machine learning technologies not only prepares agricultural businesses for the challenges of tomorrow but also positions them as leaders in a continuously evolving market.
As we advance, it is clear that the intersection of technology and agriculture will redefine the landscape of the industry, yielding significant benefits for farmers, businesses, and the global community alike.
agriculture dataset for machine learning