Machine Learning for Malware Detection: A New Era in Cybersecurity
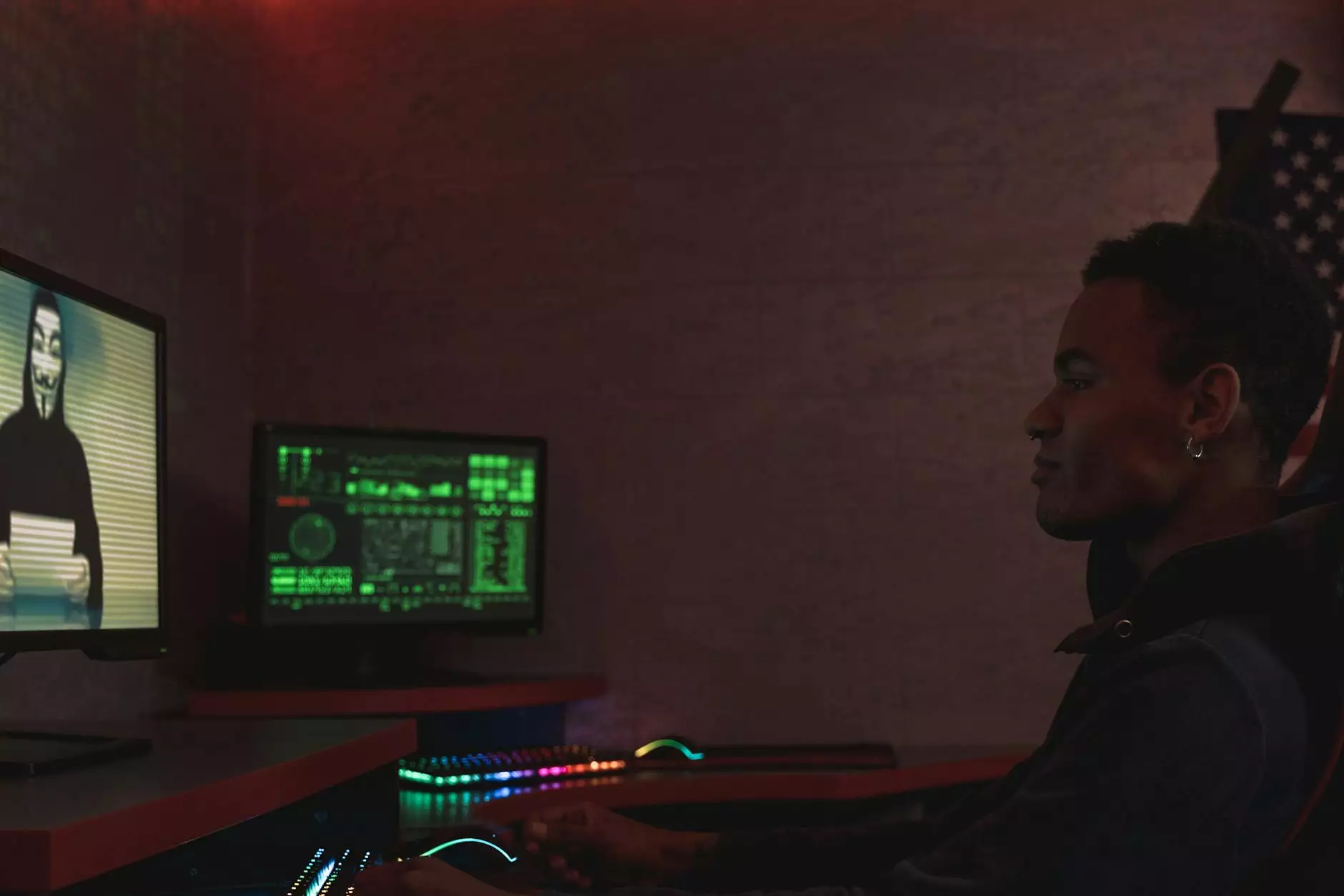
In today's digital landscape, the threat of malware is an ever-present concern for businesses and individuals alike. With the rapid evolution of technology and methodologies used by cybercriminals, traditional security measures are often insufficient. This is where machine learning for malware detection comes into play, offering innovative and effective solutions to combat these threats.
Understanding Malware and Its Impact on Businesses
Malware, short for malicious software, includes viruses, worms, spyware, and ransomware designed to disrupt, damage, or gain unauthorized access to computer systems. The ramifications of malware attacks can be severe, leading to:
- Financial Loss: Data breaches can cost businesses thousands, if not millions, in recovery and mitigation efforts.
- Reputation Damage: A single security breach can tarnish a company's reputation, eroding customer trust.
- Operational Disruption: Malware can disrupt services, affect productivity, and hinder business continuity.
- Legal Repercussions: Failure to protect sensitive data may lead to legal issues and compliance penalties.
The Role of Machine Learning in Cybersecurity
Machine learning is a branch of artificial intelligence that enables computers to learn from data and improve their performance over time without being explicitly programmed. In cybersecurity, this technology is transforming the way malware detection is approached. Here’s how:
1. Advanced Threat Detection and Classification
One of the primary advantages of machine learning for malware detection is its ability to analyze vast amounts of data quickly. Machine learning algorithms can learn from historical data to identify patterns and anomalies that might indicate a malware attack. This includes:
- Behavioral Analysis: Instead of relying solely on known malware signatures, machine learning systems can analyze the behavior of applications in real-time to identify malicious activities.
- Feature Extraction: Machine learning models can extract important features from files (e.g., byte patterns, API calls) that can help classify whether a file is benign or malicious.
2. Improvement Over Time
As these systems are exposed to more data and real-world attacks, they can refine their algorithms and improve their detection rates. This means:
- Adaptive Learning: Machine learning models adapt to new attack vectors that may not have been present during their initial training phase.
- Continuous Learning: By continuously updating the models with new data, businesses can stay ahead of emerging threats.
Implementing Machine Learning for Malware Detection
While the benefits of integrating machine learning into malware detection strategies are clear, implementing these solutions requires careful planning. Here are some key steps to ensure successful integration:
1. Identify Business Needs
Begin by assessing your organization's unique security challenges and requirements. Understanding your specific needs will help tailor the machine learning solution to provide maximum efficacy. Consider:
- Type of data being protected
- Current security measures and their effectiveness
- Regulatory compliance considerations
2. Choose the Right Tools and Technologies
The market is saturated with various tools and software for machine learning-based malware detection. When choosing the right solutions, consider:
- Reputation and Support: Select products from reputable vendors known for their cybersecurity solutions.
- Integration Capabilities: Ensure the solution can seamlessly integrate with your existing IT infrastructure.
- Performance Metrics: Look for tools that provide clear metrics to measure performance and effectiveness.
3. Train Your Staff
Even the most advanced technology is ineffective without knowledgeable personnel. Train your IT and security teams on how to leverage machine learning tools effectively. This includes:
- Understanding Machine Learning Concepts: Provide education on the fundamentals of machine learning.
- Interpreting Data: Teach them how to analyze and interpret results generated by machine learning algorithms.
4. Continuous Monitoring and Improvement
Machine learning systems require ongoing evaluation and improvement. Establish a process for:
- Regularly Updating Models: Implement a routine schedule for reviewing and updating the machine learning models you're using.
- Assessing Performance: Use analytics to assess the effectiveness of the machine learning system regularly.
Case Studies: Success Stories of Machine Learning in Action
Several organizations have successfully implemented machine learning for malware detection, showcasing the technology's potential. Here are a couple of notable examples:
1. Company A: Retail Sector
A leading retail company faced increasing instances of data breaches. By integrating a machine learning-based malware detection solution, they were able to:
- Reduce malware detection time by 75%, allowing for quicker incident response.
- Decrease the number of false positives, enabling IT staff to focus on genuine threats.
2. Company B: Financial Services
A financial institution adopted machine learning to enhance its fraud detection capabilities. The implementation resulted in:
- Identifying suspicious transactions with over 90% accuracy within minutes of detection.
- Improving compliance with regulatory standards by enhancing data protection measures.
Challenges and Considerations
While machine learning for malware detection presents numerous advantages, there are challenges to be mindful of:
1. Data Privacy Concerns
Handling sensitive data requires adhering to strict privacy regulations. Businesses must ensure that their machine learning systems comply with laws like GDPR and CCPA.
2. Model Bias
If the training data is biased, the machine learning models may produce skewed results. Regular audits and diverse datasets are essential to mitigate this risk.
3. Evolving Cyber Threats
Cybercriminals continuously adapt their tactics, meaning machine learning models must also be flexible and regularly updated to respond effectively to new threats.
Conclusion: Future of Malware Detection
As cyber threats continue to evolve, the role of machine learning for malware detection will only grow more significant. By integrating machine learning technologies into cybersecurity strategies, businesses can protect themselves from malicious actors more effectively. With ongoing advancements, businesses equipped with these tools will not only survive but thrive in the complex digital landscape.
Join the Movement to Enhance Cybersecurity
At spambrella.com, we recognize the importance of robust cybersecurity measures. Partner with us to explore cutting-edge IT Services & Computer Repair and Security Systems that leverage the power of machine learning. Together, we can safeguard your business against the ever-present threat of malware and ensure your digital environment remains secure.